Auto-GPT vs AgentGPT: Understanding the Differences In 2023? In the ever-evolving landscape of artificial intelligence (AI) and natural language processing (NLP), two prominent language models have garnered significant attention: Auto-GPT and AgentGPT.
These advanced AI systems have revolutionized the way we interact with machines and have found applications in various domains. In this article, we will delve into the nuances of Auto-GPT and AgentGPT, exploring their capabilities, use cases, and the key differences that set them apart.
Understanding Auto-GPT
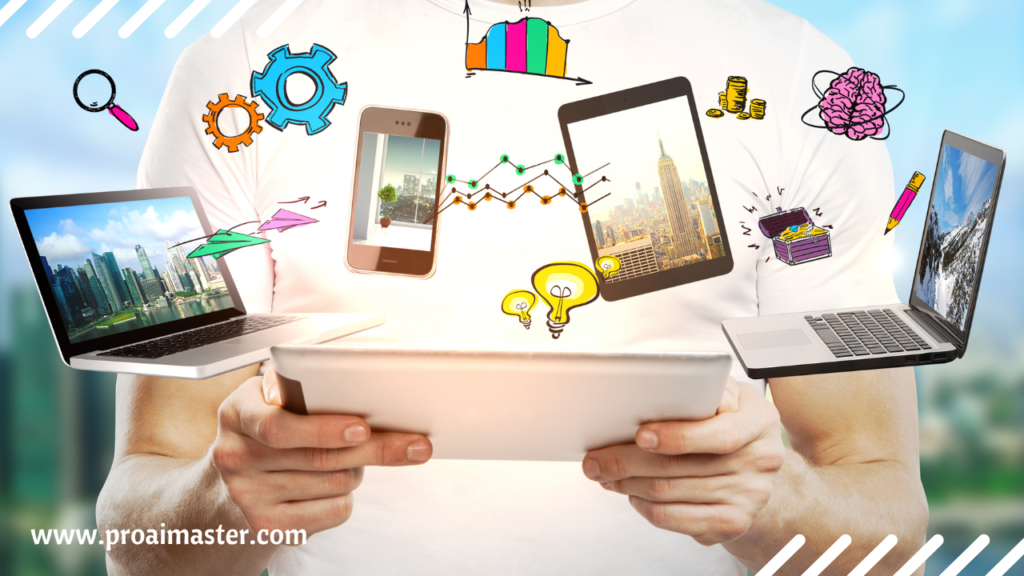
Auto-GPT, short for “Automated Generative Pre-training Transformer,” is an AI model developed by OpenAI. It is a state-of-the-art language model based on the GPT architecture, equipped with advanced machine learning techniques.
Auto-GPT is designed to generate coherent and contextually relevant responses given a prompt or question. Its training involves pre-training on a massive corpus of text data to develop a deep understanding of language patterns, grammar, and context.
Auto-GPT serves as a versatile tool for various applications such as content generation, text completion, chatbots, and even aiding in code writing. It can understand the user’s intent and provide relevant information or assistance in a conversational manner. The model’s ability to generate human-like responses has made it popular for tasks that require natural language understanding and generation.
Unveiling AgentGPT
AgentGPT, on the other hand, is an extension of Auto-GPT specifically designed to excel in interactive and dynamic environments. While Auto-GPT focuses on generating responses based on a given prompt, AgentGPT goes a step further by actively engaging in dialogues with users and other AI agents.
AgentGPT is built with reinforcement learning techniques, allowing it to learn from its interactions and improve its responses over time. It is trained in a simulated environment where it plays both user and agent roles, refining its conversational abilities. The agent-environment interplay fosters an adaptive and context-aware nature in AgentGPT, enabling it to hold more coherent and dynamic conversations.
The Role of Context in Auto-GPT and AgentGPT
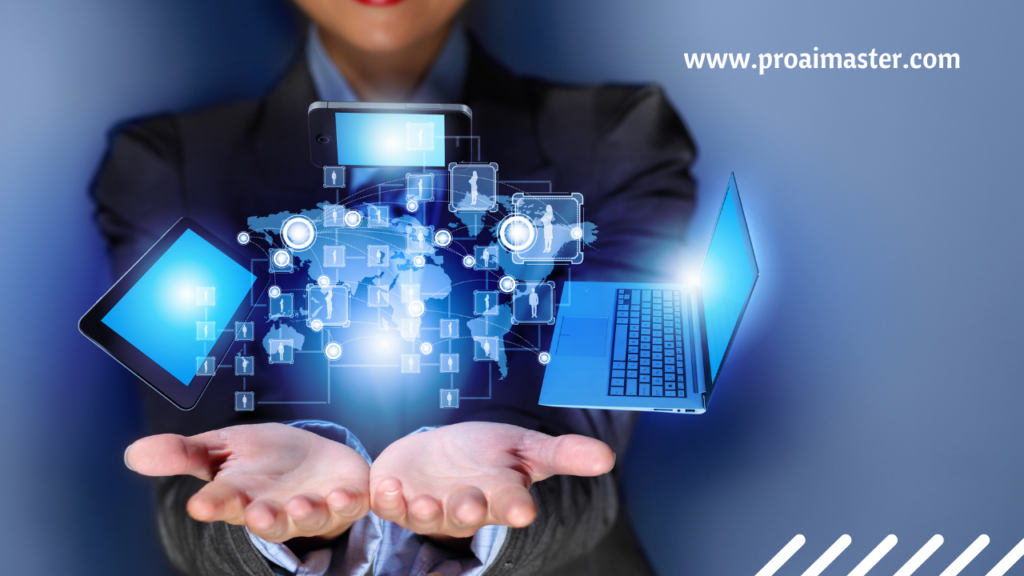
One crucial distinction between Auto-GPT and AgentGPT lies in their treatment of context. Auto-GPT primarily focuses on generating responses based on the immediate context of the given prompt. It does not possess an explicit memory of previous interactions or dialogue history, resulting in context limitations.
In contrast, AgentGPT is specifically designed to handle context-rich conversations. It maintains an internal memory that retains previous dialogue history and uses it to generate more contextually appropriate responses. This contextual understanding allows AgentGPT to engage in longer and more coherent conversations, with a stronger sense of continuity and relevance.
Applications and Use Cases
Both Auto-GPT and AgentGPT have wide-ranging applications across numerous industries. Auto-GPT’s natural language processing capabilities find use in content creation, writing assistance, customer support chatbots, and even as creative writing aids. Its ability to generate human-like text has proven invaluable for tasks where generating coherent and contextually accurate responses is paramount.
AgentGPT’s enhanced conversational abilities make it a suitable choice for interactive and dynamic applications. It can be employed in virtual assistants, customer service chatbots, and multi-agent environments, facilitating seamless and contextually rich conversations with users. AgentGPT’s adaptive nature allows it to learn from interactions, ensuring continual improvement in its dialogue skills.
Key Differences and Limitations
The key differences between Auto-GPT and AgentGPT lie in their primary focus and capabilities. Auto-GPT excels in generating text based on a given prompt but lacks explicit memory and context awareness. It is ideal for single-turn tasks where continuity and context are not critical.
AgentGPT, however, shines in multi-turn conversations. With its ability to retain context and generate dynamic responses, it is better suited for tasks that require extended interactions and a deeper understanding of conversation history. Nonetheless, both models have limitations. They may produce plausible-sounding but incorrect or biased answers, and their responses are influenced by the data on which they are trained.
Training Methodologies:
Auto-GPT is trained using unsupervised learning techniques, where it learns from a large dataset of text by predicting the next word in a sentence. This process helps it capture grammar, syntax, and contextual patterns.
On the other hand, AgentGPT is trained using a combination of supervised and reinforcement learning. It leverages both human-generated dialogues and simulated interactions to learn how to engage in dynamic conversations effectively.
Contextual Understanding:
While Auto-GPT focuses on generating responses based on the immediate context of the prompt, AgentGPT takes context into account throughout the conversation.
AgentGPT’s ability to retain and recall previous dialogue history allows it to provide more coherent and relevant responses, creating a more engaging and seamless conversational experience.
Adaptability:
AgentGPT is designed to be adaptive and learn from its interactions. It employs reinforcement learning techniques to improve its responses over time based on feedback from users or other AI agents.
This adaptability makes AgentGPT well-suited for evolving conversations and enables it to handle a wide range of user inputs more effectively.
Handling Ambiguity:
Both Auto-GPT and AgentGPT face challenges when it comes to ambiguous queries or prompts. However, AgentGPT’s contextual understanding and memory of previous interactions allow it to handle ambiguity more gracefully.
It can infer meaning from the context and generate responses that align with the user’s intent, even when faced with unclear or incomplete queries.
Use Cases:
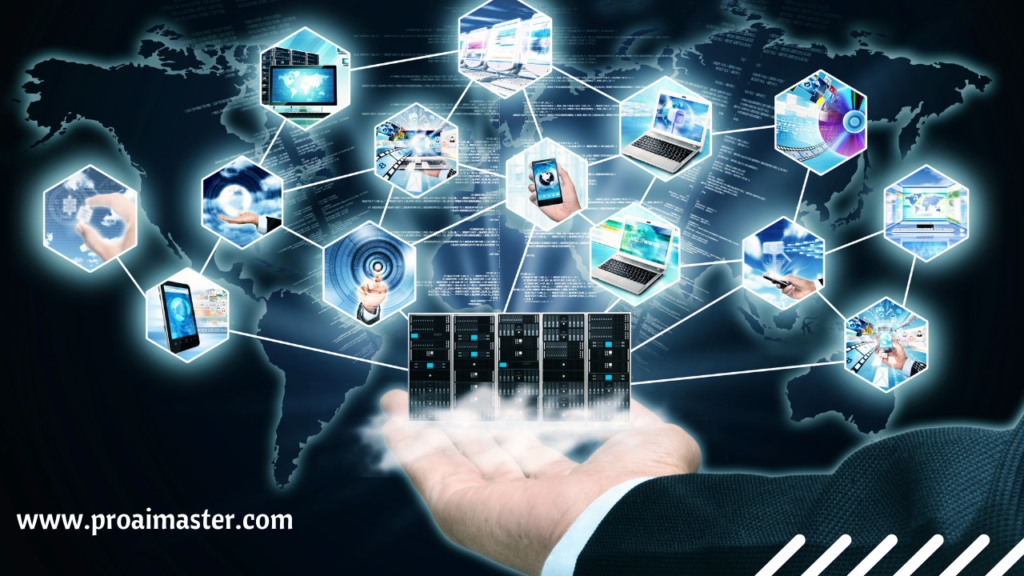
Auto-GPT finds applications in various domains, including content generation, text completion, and language translation. Its ability to produce coherent and contextually accurate responses makes it useful for tasks where generating human-like text is crucial.
AgentGPT, with its emphasis on dynamic conversations, is well-suited for virtual assistants, customer support chatbots, and gaming environments where users interact with AI agents.
Ethical Considerations:
Both Auto-GPT and AgentGPT can exhibit biased behavior or generate inappropriate content if trained on biased or offensive data.
OpenAI has implemented safeguards and ethical guidelines to mitigate such issues, but it remains an ongoing challenge. It is essential to ensure responsible and ethical use of these models to avoid unintended consequences.
Future Developments:
As AI research progresses, further advancements are expected in both Auto-GPT and AgentGPT.
Future iterations may focus on improving contextual understanding, addressing bias concerns, and enhancing the models’ ability to handle complex queries and conversations. Continued research and development in this field will lead to more sophisticated and human-like AI language models.
Conclusion
In summary, Auto-GPT and AgentGPT are two advanced AI language models that have revolutionized the field of natural language processing. While Auto-GPT focuses on generating text based on a given prompt, AgentGPT goes beyond by actively engaging in dynamic conversations.
Understanding their differences and capabilities allows us to leverage their strengths in different scenarios. As AI technology continues to evolve, these models pave the way for more intelligent and contextually aware systems that enhance human-machine interactions.
FAQs
What is the difference between Auto-GPT and AgentGPT?
Auto-GPT is a language model that focuses on generating text based on a given prompt, while AgentGPT is an extension that is designed for dynamic and context-rich conversations. AgentGPT actively engages in dialogues, retains context, and learns from interactions, allowing for more coherent and adaptive responses.
What are the main applications of Auto-GPT and AgentGPT?
Auto-GPT finds applications in content generation, text completion, chatbots, and code writing assistance. It is useful in tasks that require generating human-like text. AgentGPT is suitable for virtual assistants, customer support chatbots, and multi-agent environments, where interactive and dynamic conversations are essential.
How do Auto-GPT and AgentGPT handle context in conversations?
Auto-GPT generates responses based on the immediate context of the prompt, without an explicit memory of previous interactions. AgentGPT, however, maintains a memory of dialogue history, allowing it to generate more contextually appropriate responses and engage in longer and more coherent conversations.
Can Auto-GPT and AgentGPT handle ambiguous queries or prompts?
While both models face challenges with ambiguity, AgentGPT’s contextual understanding and memory of previous interactions enable it to handle ambiguous queries more effectively. It can infer meaning from the context and generate responses that align with the user’s intent.
What are the limitations of Auto-GPT and AgentGPT?
Auto-GPT and AgentGPT may produce plausible-sounding but incorrect or biased answers, as their responses are influenced by the data they are trained on. They may also struggle with complex or nuanced queries, and ethical concerns related to bias and inappropriate content generation need to be considered.
How are Auto-GPT and AgentGPT trained?
Auto-GPT is trained using unsupervised learning techniques, where it learns from a large dataset of text by predicting the next word in a sentence. AgentGPT, on the other hand, is trained using a combination of supervised and reinforcement learning, utilizing both human-generated dialogues and simulated interactions.
Can Auto-GPT and AgentGPT be improved in the future?
Yes, ongoing research and development in the field of AI will lead to further advancements in Auto-GPT and AgentGPT. Future iterations may focus on improving contextual understanding, addressing bias concerns, enhancing the ability to handle complex queries, and refining the models’ overall performance.
What are the ethical considerations when using Auto-GPT and AgentGPT?
It is important to ensure responsible and ethical use of Auto-GPT and AgentGPT. These models can exhibit biased behavior or generate inappropriate content if trained on biased or offensive data. OpenAI and other organizations are actively implementing safeguards and ethical guidelines to mitigate such issues.
Can Auto-GPT and AgentGPT understand and process different languages?
Yes, Auto-GPT and AgentGPT have the ability to understand and process multiple languages. They can be trained on diverse datasets and can be used for various language-related tasks across different linguistic contexts.
How can businesses benefit from using Auto-GPT and AgentGPT?
Yes, Auto-GPT and AgentGPT have the ability to understand and process multiple languages. They can be trained on diverse datasets and can be used for various language-related tasks across different linguistic contexts.
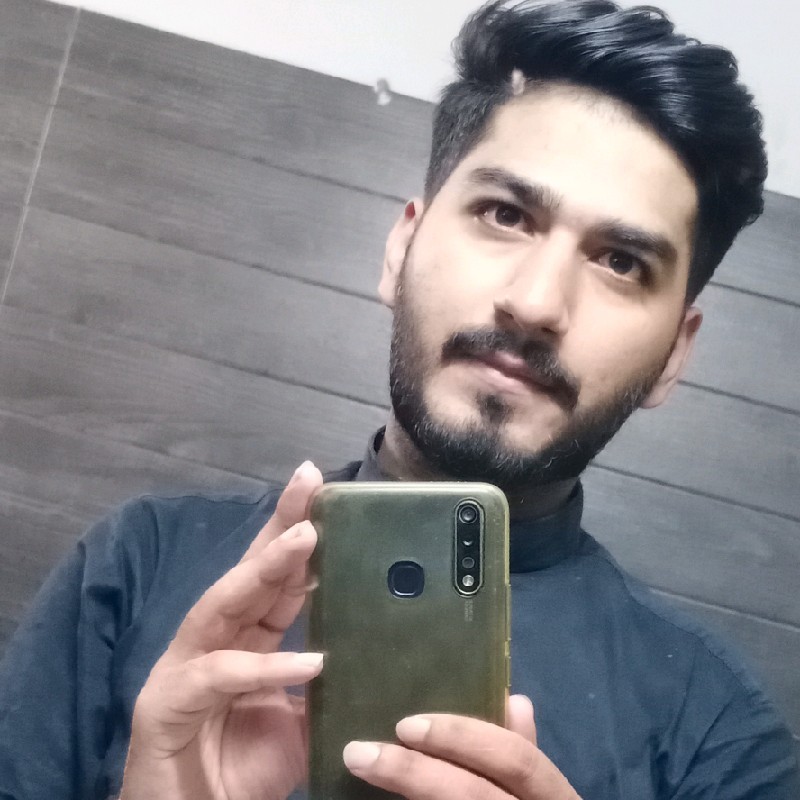
Muhammad Ahmad is a dedicated writer with 5+ years of experience delivering engaging and impactful content. He specializes in simplifying complex topics into easy-to-read articles.