How to Fix “ChatGPT is at Capacity Right Now”. ChatGPT, powered by the remarkable GPT-3.5 language model, has become an invaluable tool for countless users seeking assistance, information, or even just engaging in conversations.
However, due to its popularity and demand, ChatGPT occasionally reaches its capacity, leading to frustration and inconvenience for users.
In this article, we will explore why ChatGPT may experience capacity issues and provide some potential solutions to address this challenge.
Understanding ChatGPT’s Capacity Limitations:
ChatGPT operates within finite resources, including computational power and memory. These limitations can result in the system reaching its capacity and being unable to accept new requests or provide prompt responses. Such circumstances often arise during periods of high user traffic or overwhelming demand.
Causes of “ChatGPT is at Capacity Right Now”:
There are several factors that can contribute to ChatGPT reaching its capacity:
- User Load: When numerous users concurrently access ChatGPT, it can strain the system’s available resources, leading to slower response times or capacity overload.
- Complex or Lengthy Interactions: Some conversations require extensive context and involve multiple back-and-forth exchanges. These complex interactions consume more computational resources, potentially exhausting ChatGPT’s capacity faster.
- Resource Allocation: ChatGPT’s capacity is also influenced by the allocation of computational resources across multiple applications and user groups. If resource allocation is not optimized, it can impact the overall capacity of the system.
Solutions to Address Capacity Issues:
To mitigate capacity overload in ChatGPT, several potential solutions can be explored:
- Scalability: Enhancing ChatGPT’s infrastructure by deploying additional computational resources and optimizing resource allocation can help improve its capacity to handle a larger user load.
- Load Balancing: Implementing load balancing techniques can distribute user requests across multiple instances of ChatGPT, ensuring more efficient utilization of available resources and reducing the likelihood of capacity overload.
- Prioritization and Queuing: Introducing a prioritization system that categorizes user requests based on urgency or importance can help manage capacity issues. By queuing requests during peak times and gradually processing them when resources become available, a fair and efficient distribution of responses can be achieved.
- Context Optimization: Users can contribute to capacity optimization by structuring their inquiries to be more concise and specific. By providing relevant information upfront and avoiding unnecessary conversation threads, users can help reduce the computational load on ChatGPT.
- Enhanced Resource Management: Continual monitoring and analysis of user traffic patterns, computational resource usage, and response times can assist in identifying bottlenecks and optimizing resource allocation accordingly. Machine learning algorithms can aid in predicting demand spikes and preemptively allocating resources to mitigate capacity issues.
Communication and Transparency:
During periods of capacity overload, maintaining transparent communication with users is crucial. Proactive notifications and status updates can inform users about potential delays or temporary unavailability, setting appropriate expectations and reducing frustration.
Additionally, providing alternative channels or fallback options, such as human-assisted support, can ensure users have access to assistance when ChatGPT reaches its capacity.
Hybrid Approach:
To address capacity issues, a hybrid approach can be considered. This involves combining the power of AI-driven language models like ChatGPT with human assistance.
By seamlessly integrating human agents into the system, complex or critical queries can be efficiently handled, offloading some of the computational burdens from ChatGPT and ensuring prompt and accurate responses.
Preemptive Scaling:
By closely monitoring usage patterns and analyzing historical data, developers can proactively scale up ChatGPT’s capacity during periods of anticipated high demand.
Predictive analytics and machine learning algorithms can assist in identifying patterns and predicting future traffic spikes, enabling the system to dynamically adjust its resources to meet user needs.
Research and Development:
Continuous research and development efforts aimed at improving the efficiency and scalability of language models can contribute to addressing capacity issues.
Advancements in model architecture, optimization techniques, and resource management algorithms can lead to more robust and scalable systems, ensuring smoother user experiences even during peak usage periods.
User Education:
Educating users about the limitations and capabilities of ChatGPT can help manage expectations and reduce frustration. Providing guidance on structuring queries effectively, avoiding unnecessary interactions, and utilizing alternative channels during peak times can empower users to optimize their interactions with the system and contribute to overall capacity management.
Collaborative Efforts:
Capacity challenges are not unique to ChatGPT alone. Engaging in collaborative efforts with researchers, developers, and industry stakeholders can lead to the sharing of best practices, collective knowledge, and innovative solutions.
Open dialogue and collaboration can help address capacity issues not only for ChatGPT but for other AI-driven systems as well.
Future Prospects:
As technology progresses, we can expect advancements in AI infrastructure and distributed computing, which will likely contribute to improved capacity management.
Additionally, ongoing research on more efficient language models and novel approaches to conversation processing will further enhance the scalability and responsiveness of systems like ChatGPT.
Conclusion:
ChatGPT’s occasional capacity overload is a testament to its immense popularity and usefulness. By understanding the underlying causes and implementing suitable solutions, developers can enhance the system’s capacity and improve user experience.
Through scalability, load balancing, prioritization, context optimization, and enhanced resource management, ChatGPT can continue to serve users efficiently while minimizing capacity-related disruptions.
Striving for transparency and maintaining effective communication during peak times is equally essential to ensure users feel informed and supported. As technology advances, we can anticipate continued improvements in capacity management, leading to even more reliable and seamless interactions with ChatGPT in the future.
FAQs
Why does ChatGPT reach its capacity?
ChatGPT operates within finite computational resources, including memory and processing power. When there is a high influx of user requests or complex interactions that require extensive computational resources, ChatGPT can reach its capacity and experience delays or temporarily become unavailable.
How can I avoid ChatGPT’s capacity issues?
To help mitigate capacity issues, you can structure your queries to be concise and specific, providing relevant information upfront. Avoiding unnecessary conversation threads and optimizing the context of your interactions can reduce the computational load on ChatGPT. Additionally, being aware of peak usage times and considering alternative channels or fallback options when ChatGPT is at capacity can help ensure a smoother experience.
What can developers do to address capacity overload?
Developers can implement various strategies to tackle capacity overload. These include scalability enhancements, such as deploying additional computational resources and optimizing resource allocation. Load balancing techniques can also be employed to distribute user requests across multiple instances of ChatGPT. Prioritization and queuing systems can manage requests during peak times, while enhanced resource management and continuous monitoring can aid in identifying bottlenecks and optimizing resource allocation.
How can human assistance be integrated with ChatGPT?
A hybrid approach that combines AI-driven language models like ChatGPT with human assistance can be adopted. By seamlessly integrating human agents into the system, complex or critical queries can be efficiently handled, reducing the computational burden on ChatGPT and ensuring prompt and accurate responses.
Is there a solution in development to overcome capacity issues?
Ongoing research and development efforts are focused on improving the efficiency and scalability of language models like ChatGPT. Advancements in model architecture, optimization techniques, and resource management algorithms are being explored to address capacity issues. Additionally, technological advancements in AI infrastructure and distributed computing are expected to contribute to improved capacity management in the future.
What can users expect during periods of capacity overload?
During capacity overload, users may experience slower response times, delays in receiving responses, or temporary unavailability of ChatGPT. To manage expectations, transparent communication from developers, including proactive notifications and status updates, can inform users about potential delays and alternative options for assistance.
Will capacity management improve in the future?
As technology advances, we can anticipate continual improvements in capacity management for AI-driven conversational agents. Ongoing research, collaborative efforts, and advancements in AI infrastructure are likely to result in more robust and scalable solutions, ensuring reliable and seamless user experiences even during peak usage periods.
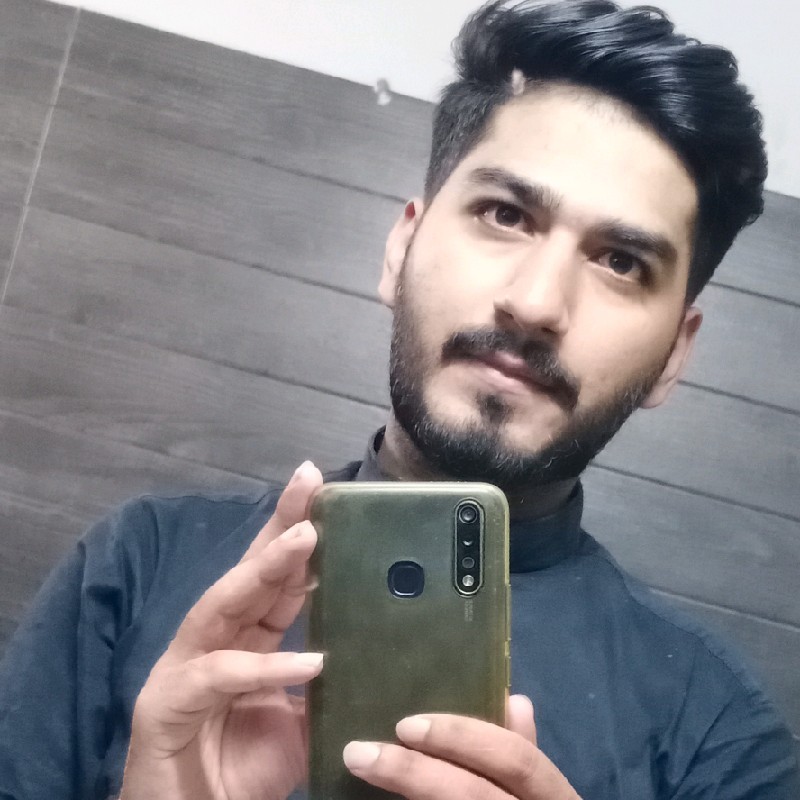
Muhammad Ahmad is a dedicated writer with 5+ years of experience delivering engaging and impactful content. He specializes in simplifying complex topics into easy-to-read articles.